PhD student:
Andrea Pozo Estivariz (PhD student, UoC)
Supervisors:
Emily Lane (NIWA)
Matthew Wilson (UoC)
Marwan Katurji (UoC)
Fernando Méndez (Universidad de Cantabria)
Funding:
MBIE Endeavour Research Programme – Increasing flood resilience across Aotearoa, New Zealand.
Summary:
Flooding is the most frequent natural hazard in New Zealand and the second-most costly after earthquakes. It is also expected to become more severe as climate change impacts are realized. Generally, two-dimensional hydrodynamics models are used to simulate flood inundation across a floodplain. These models can provide reliable estimations of the flood extent and magnitude. However, they are also computationally expensive and time-consuming. Current computing power and memory pose a limitation in the number, level of detail and modelling time of assessed flooding scenarios, making rapid real-time or forecast flooding very challenging. This work investigates the application of hybrid hydrodynamic and machine learning (ML) techniques to develop a rapid flood scenario assessment model using the Wairewa catchment (Banks Peninsula, Canterbury) as the study site. This approach aims to reduce the numerical modelling load and enable probabilistic modelling, allowing us to make rapid predictions of potential flooding events from an ensemble of previously assessed events. The hybrid model is able to predict flood extent and maximum flood depth based on the characteristics of the main inundation driver in the area (heavy rainfall) and of the geographic features of the catchment. To do this, firstly, a sample of synthetic storms was created based on the temporal and spatial characteristics of the heavy rainfall events in the catchment. Then, each storm in this sample was modelled using a 2D hydrodynamic model, BG-Flood, to obtain the corresponding flood extent and maximum water depth map. Following this, the sample of storms (with their corresponding inundation maps) was divided into training, validation and testing datasets to experiment with different ML algorithm. Random Forest (RF) is capable of predicting flood extent and maximum water depth in the floodplain of the catchment very well. In the ongoing work a hybrid hydrodynamic and deep learning (DL) model is being built focusing in generating inundation maps at finer spatial resolution (5 m) and in less time.
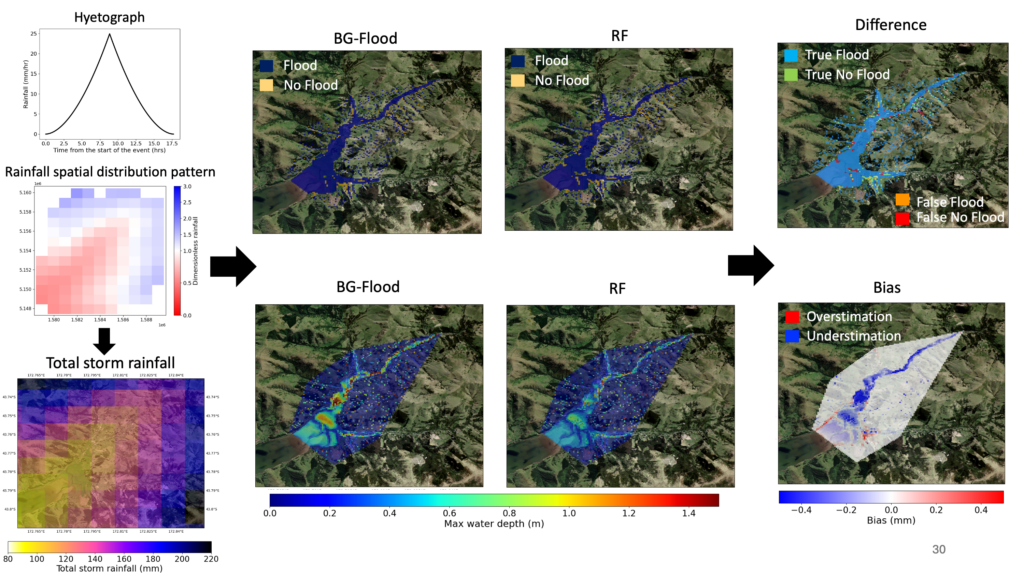
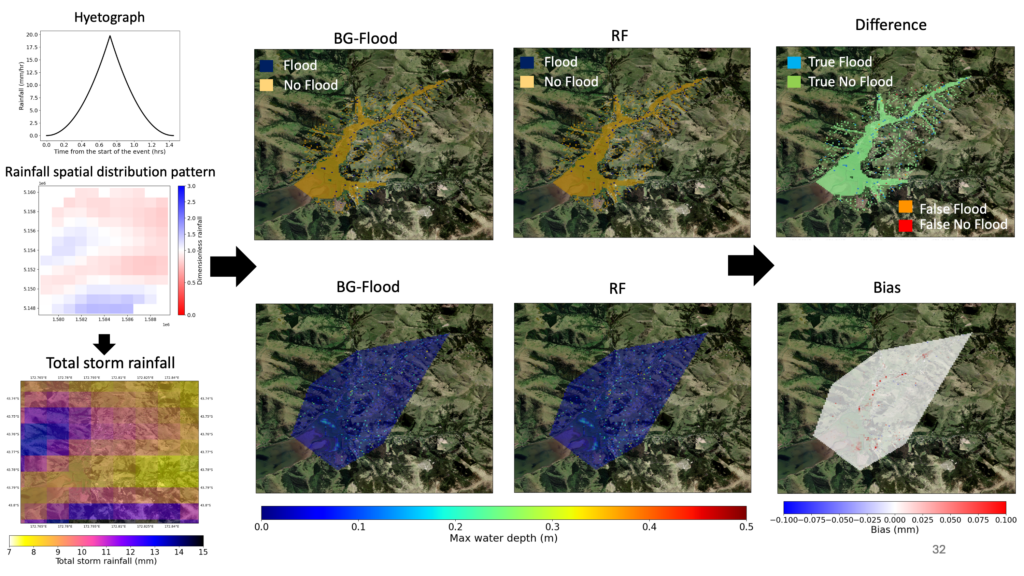
Outputs:
Pozo, A., Wilson, M., Lane, E., Méndez, F. and Katurji, M. (2023). Towards a method of rapid flood scenario mapping using hybrid approaches of hydraulic modelling and machine learning, EGU General Assembly 2023, Vienna, Austria, 24–28 Apr 2023, EGU23-2462, https://doi.org/10.5194/egusphere-egu23-2462.
Pozo, Andrea. Cross validation of characterisation of rainfall extreme events between rain gauge data and WRF model in North Canterbury. Waterways Postgraduate Students Conference, Christchurch, 8 November 2022.
Pozo, A., Wilson, M., Katurji, M., Méndez, F. and Lane, E. Analysis of New Zealand Daily Weather Patterns (DWTS) and Large-Scale Climatic Patterns as Extreme Rainfall and Flooding Drivers. The Fourth Spatial Data Science Symposium. UoC, Christchurch, September 5, 2023. Poster.
Pozo, A. Analysis of New Zealand daily weather patterns (DWTs) and large scale climatic patterns as heavy rainfall and flooding drivers. Australasian Groundwater & New Zealand Hydrological Society Joint Conference. Auckland, December 2023.