Project lead: Dr. Emily Lane (NIWA)
GRI Team: Prof. Matthew Wilson (Uncertainty Lead)
Dr Rose Pearson (NIWA/GRI visiting research fellow)
Martin Nguyen (UoC PhD student)
Andrea Pozo Estivariz (UoC PhD student)
Katherine Booker (UoW PhD student)
Clevon Ash (UoC PhD student)
Funding: MBIE Endeavour Research Programme – Increasing flood resilience across Aotearoa, New Zealand.
Summary:
Flooding is one of New Zealand’s most damaging hazards, which will also change the most rapidly in intensity and nature as a result of climate change. At the same time, urban development is leading to increased flood exposure. These dual challenges make reducing flood risk extremely difficult for our current planning and response systems. There is a knowledge vacuum about the scale of these problems, the integration of different policy domains, and the details of how different parts of the country will be affected. In this 5-year programme, we are working in partnership with NIWA and other organisations including universities, CRIs, local and central government and Iwi to support the changes that are needed to improve our management of flood risk. In particular, we will help to produce New Zealand’s first consistent national flood map, showing where flooding is likely to occur and the impacts associated with it.
The primary focus of our work will be to quantify the uncertainties associated with these predictions, particularly those which result from the representation of spatial data within hydraulic modelling. We will assess how uncertainty “cascades” through the modelling/ analysis chain, enabling us to determine our level of confidence with projected flood risk. This will provide policy-makers with the information needed to be able to make decisions despite uncertainty in scientific predictions. Uncertainty is a cross-cutting theme that will assess the drivers and consequences of uncertainty and work with end-users to design, test and establish novel decision-making practices.
Uncertainties in flood risk assessments
Prof. Matt Wilson is leading the cross-programme uncertainty theme for Mā te Haumaru ō te Wai. This research aims to advance our understanding and handling of the uncertainties which are present in predictions of flood inundation. Flood risk and other planning practitioners worldwide often use the outputs from flood modelling as part of their decision-making, such as when they determine flood hazard zones, design mitigation measures, or assess the potential impacts of climate change on the flood hazard. However, the uncertainty in these outputs is not often quantified or characterised, making the decision-making process more challenging and less reliable.
To account for uncertainty, planners may take a precautionary approach, such as adding a freeboard amount to required floor levels in flood zones or designing flood infrastructure such as stopbanks (levees) to a 1% annual exceedance probability (i.e., the 100-year average recurrence interval). However, this approach is questionable in an era of changing risk under climate change. For example, is the freeboard amount used sufficient to prevent serious damage from future floods? Will the area at flood risk increase? Will a current 100-year flood become a 50-year flood in future?
Some of these questions are aleatoric in nature: they will always be present and cannot be reduced. This includes issues such as the internal variability of the climate system, the implication of which is that, even if we had complete information about the future climate state, its chaotic nature means our flood risk assessments will still be uncertain. Other uncertainties are epistemic and are deterministic and subjective; the uncertainty contained in a flood risk assessment depends on how good (or bad!) are the data which are used within the analysis. Improving input data accuracy and model representations should, at least theoretically, reduce the inherent uncertainty in the predictions obtained and is something we always aim for.
Yet, even if we use the best possible data and model representations, uncertainty will still result from a complex combination of errors associate with source data, sampling and model representation – uncertainties which “cascade” through the risk assessment system (see right), reducing our confidence in any individual prediction and leading to variability in predicted depths and extents across multiple predictions which account for these errors (e.g., within Monte Carlo analysis). These uncertainties, here represented as variability in predicted depths and flows, further cascade through to the analysis of flood impacts.
Uncertainty in predicted depths and flows combine with errors from data such as those for buildings and infrastructure, and the statistical models used to quantify damage (e.g., via depth-damage curves). The end result is uncertainty in quantified damage for a flood scenario, creating issues for the decision-making processes such as determining whether to invest in improved mitigation measures.
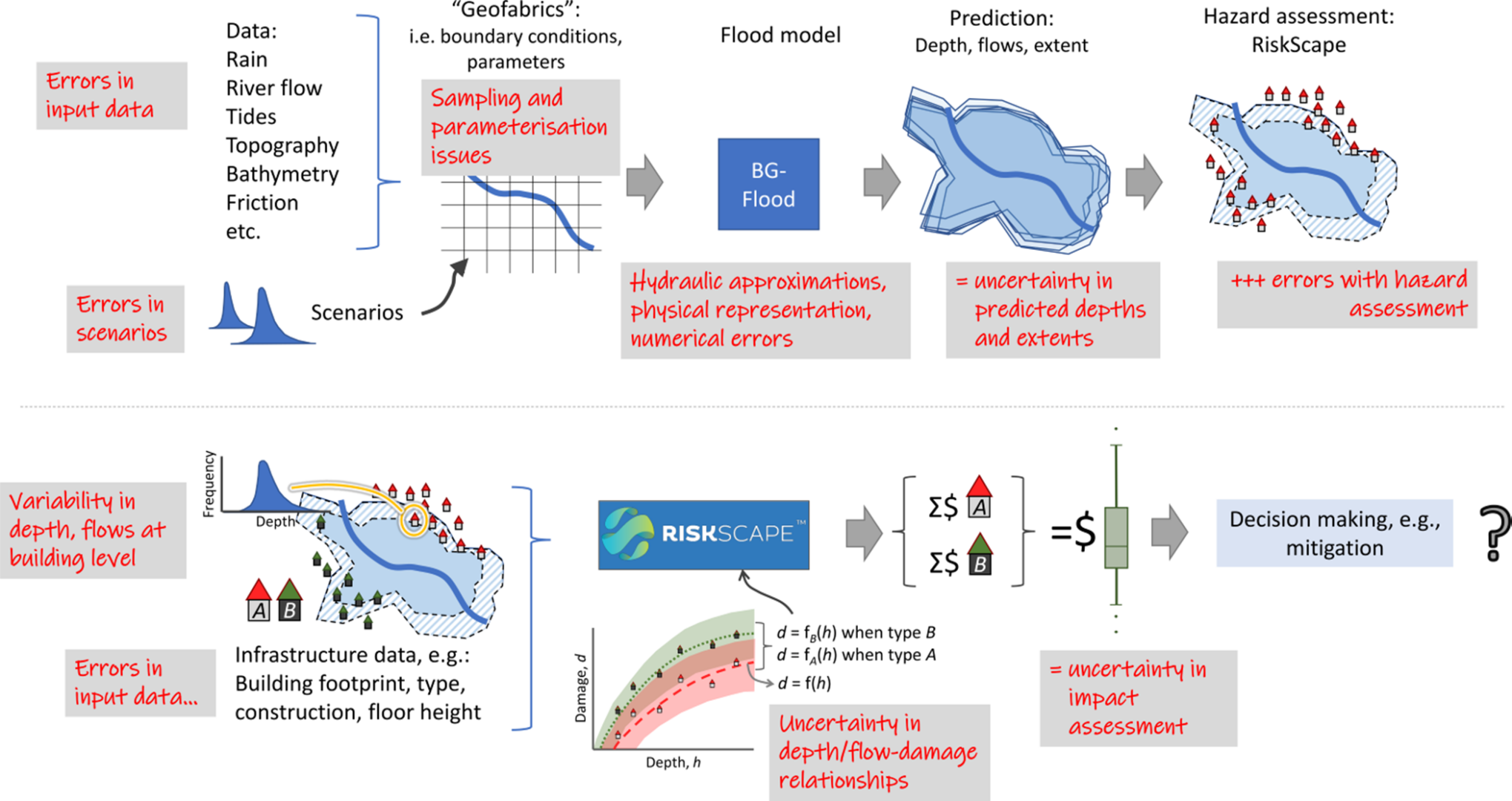
Data processing for flood risk assessments
Alongside the uncertainty research, the GRI is working with NIWA to help produce consistent, open-source methodology for the national flood risk assessments. The methods developed are guided by a number of physically-based flood inundation modelling efforts that have been attempted internationally both at national and global scale. The research programme is specifically addressing common problems, including: the mismatch between resolution, accuracy and the detail required for local-level decision-making; the lack of integration of coastal, fluvial (river) and pluvial (rainfall) hazards; poor integration with urban development futures; and an inadequate representation of uncertainty.
GRI Visiting Researcher Dr Rose Pearson is working towards the development of automated techniques for building a nation-wide datasets suitable for hydraulic modelling, including hydrologically-conditioned digital elevation model (DEM) and roughness maps. Automated processing is needed as these datasets evolve with time, as better survey data becomes available, and in the event of tectonic movements, erosion, earthworks and land-use changes.
An open-source Python library, “GeoFabrics”, has been developed to support the production of datasets from LiDAR and other data. In addition, another library called “geoapis” has been developed to facilitate the programmatic acquisition of the latest LiDAR and vector data available on Open Topography, LINZ LDS, LRIS, and StatsNZ LDS.
PhD Projects:
Outputs:
Nguyen, M., Wilson, M., Lane, E., and Brassington, J., Uncertainty in Predictions of Flood Inundation Caused by Model Grid Sampling: AGU Fall Meeting 2022, Chicago, 12-16 December.
Pozo, A., Wilson, M., Lane, E., Méndez, F. and Katurji, M. (2023). Towards a method of rapid flood scenario mapping using hybrid approaches of hydraulic modelling and machine learning, EGU General Assembly 2023, Vienna, Austria, 24–28 Apr 2023, EGU23-2462, https://doi.org/10.5194/egusphere-egu23-2462.
Wilson, M.D., Preston, G., Li, C., Cai, X., Pearson, R., Parkinson, L., Deakin, R. and Lane, E. Flood Resilience Digital Twin (FReD): Empowering Decision Makers for Improved Management of Flood Risk. AGU23. San Francisco, December 12, 2023. Poster.