PhD student:
Martin Nguyen (PhD student, UoC)
Supervisors:
Matthew Wilson (Main Supervisor, UoC)
Emily Lane (Supervisor, NIWA)
James Brasington (UoC)
Rose Pearson (NIWA)
Funding:
MBIE Endeavour Research Programme – Increasing flood resilience across Aotearoa, New Zealand
Summary:
Topography is an important input for flood inundation model and how to obtain accurate representations of it has not been properly studied. In general, for flood hazard assessment, Airborne LiDAR point cloud data is sampled and interpolated onto a Cartesian grid (raster) to create a Digital Elevation Model (DEM). Usually, grid alignment is not considered in the processing. However, considering orientation in sampling process may introduce variability in the resulting elevation model, leading to uncertainty that propagates through to flood model output. This may be particularly apparent for raster grid-based models, where the routing of water flow on the grid may not align with environmental features such as drainage channels.
This research investigates the variation in the outputs of a flood model using a Monte-Carlo procedure, where multiple, equally likely DEMs are derived from LiDAR by adjusting the alignment (rotation) and point of origin of the model grid, and each used to predict flood inundation.
To interpret the variability in water depth values when the model grid is transform, two major comparisons were made, The first was to compare different types of transformations and the second was to compare various DEM resolutions.
Numerous studies have extensively employed Monte Carlo-based methods to characterise uncertainty in flood modelling due to their simplicity and flexibility. However, these approaches are time-consuming and computationally extensive. Recognising the need for efficiency, machine learning has emerged as a promising alternative with comparable results.
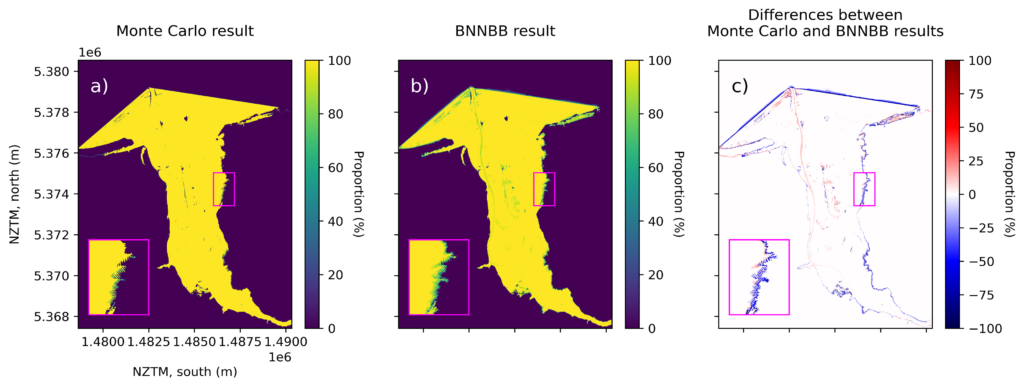
This research introduces an advanced uncertainty estimation method for flood modelling, utilizing the Bayes Neural Network with Bayes by Backprop. The model aims to forecast variations in flood modelling predictions caused by uncertainty in the Digital Elevation Model generation process, offering a more efficient and accurate approach to address the challenges posed by traditional Monte Carlo methods.
Outputs:
Nguyen, M., Wilson, M., Lane, E., and Brassington, J., Uncertainty in Predictions of Flood Inundation Caused by Model Grid Sampling: AGU Fall Meeting 2022, Chicago, 12-16 December.
Nguyen, M. Uncertainty in bathymetry estimation. The Fourth Spatial Data Symposium, Christchurch, Sept 5-6, 2023. Poster.
Nguyen, M., Wilson, M., Lane, E., Brasington, J. and Pearson, R. Uncertainty in Flood Predictions Caused by River Bathymetry Estimation. AGU23. San Francisco, December 12, 2023.